
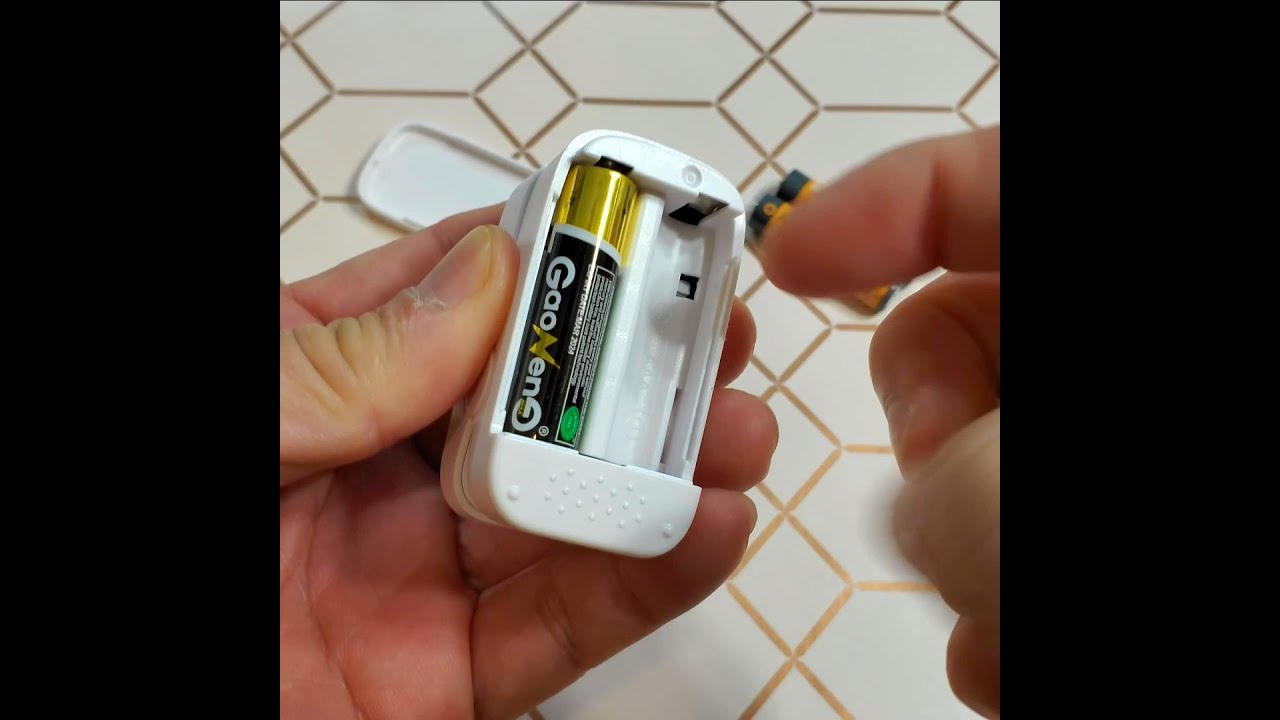
Moreover, an empirical model was proposed to estimate the capacity by describing the correlation between the aging stress factor and capacity with mathematical functions, including exponential functions, polynomial functions, and power functions. However, the inability to effectively determine batteries' cycle and calendar life under the actual duty cycle is a hurdle for practical applications. Most of the time, relevant research deals with the calendar life and cycle aging groups independently. For example, a performance-based model with a simple correlation between stress factor and capacity attenuation/impedance rise was developed, where the correlations were derived from aging tests performed under several conditions. Hence, alternative estimation methods to get a descriptive expression of battery performance levels during its entire lifetime have been proposed.
BATTERY PULSE HOW TO
Therefore, how to obtain the SOC estimation remains another challenge.

Furthermore, measurement noise from sensors could easily affect real-time resistance estimation, and capacity estimation usually relies on accurate state of charge (SOC). However, it usually takes hours to get it done with fully charging or discharging processes. The straightforward way to estimate capacity of battery is to measure its capacity directly. In the meantime, both the cyclability loss and active materials loss will reduce the battery capacity.
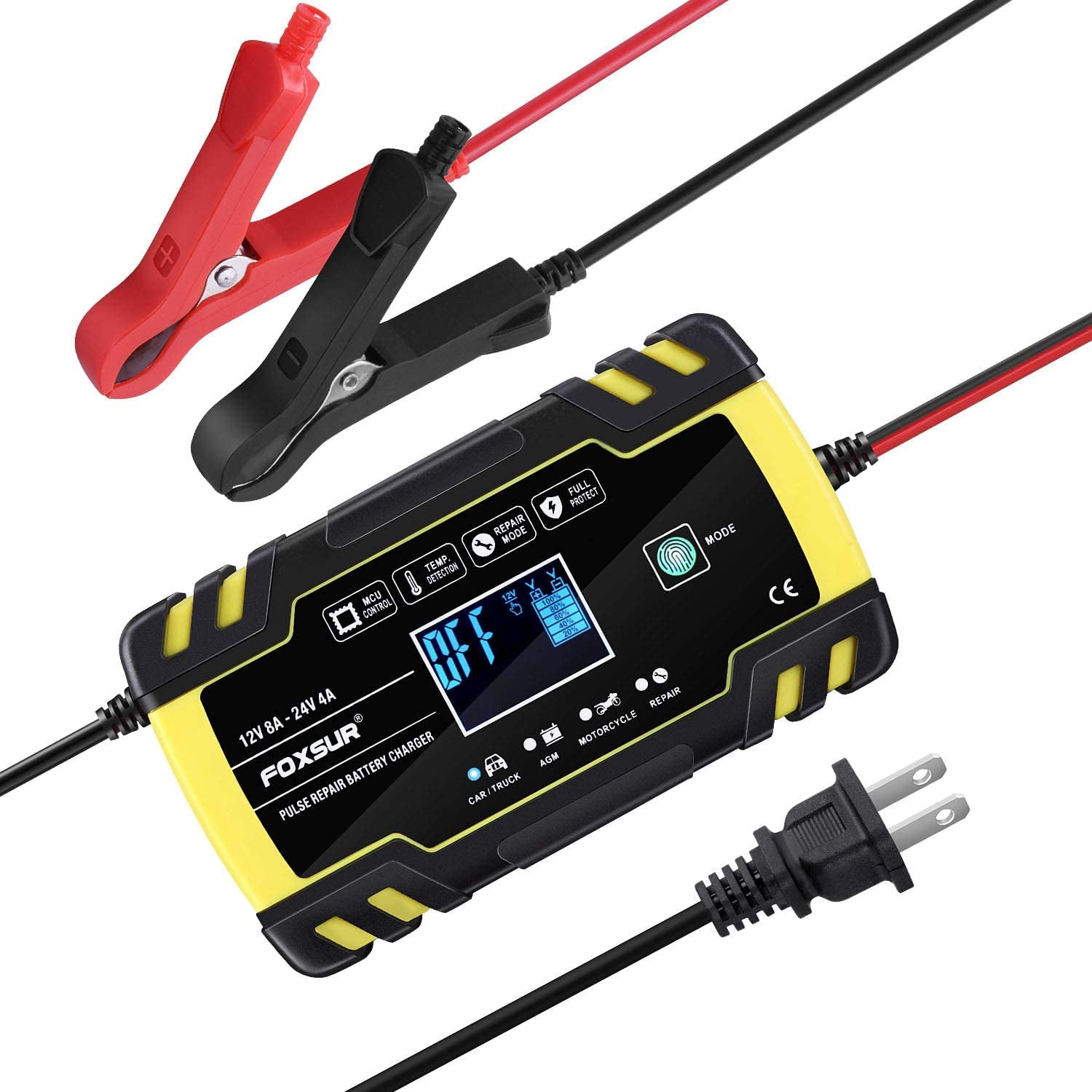
The change in electrical resistance may occur due to the passive film on the active particle surface and the losing electrical contact in the porous electrode. The secondary loss of active materials in electrodes such as material dissolution, structural degradation, particle separation, and electrode delamination. Moreover, extreme operation condition such as high temperature, overcharge, and short circuits could even deteriorate the SEI growth. The main aging factor on graphite electrodes with time is the growth of a solid interface on the electrolyte/electrode interface, which is named solid electrolyte interphase (SEI). For example, the main loss of the active lithium ions could cause battery unbalance. Various electrode-based physical mechanisms could lead to capacity loss, which can be of mechanical or chemical origin. In general, there are two major effects associated with battery aging: capacity decay and impedance increase. Especially, the battery aging is also affected by capacity decay and resistance increase.

To achieve high estimation accuracy, factors such as battery aging and degradation as well as environment or utilization patterns need to be taken into consideration. It still remains challenging to estimate their remaining capacity efficiently after tremendous batteries retire for their secondary application. Lithium-ion batteries (LIBs) have become dominant rechargeable batteries for both hybrid and plug-in EVs due to their superior performance and decreasing price. The new strategy of combining short pulse tests with machine-learning algorithms could further open window for efficiently forecasting lithium-ion battery remaining capacity.īy 2050, electric vehicles (EVs) are estimated to account for 60% of the total passenger vehicle market, with operations in all major world regions. In particular, we explore the validity of five different machine-learning methods for estimating capacity driven by pulse features, whereas Gaussian process regression with Matern kernel performs the best, providing guidance for future exploration. Data at different voltage stages and state of charge (SOC) are collected and explored to find the most suitable estimation model. The short-term features of the current pulse test were selected for an optimal training process. Interestingly, the proposed pulse test strategy for battery capacity measurement could reduce test time by more than 80% compared with regular long charge/discharge tests. Herein, by integrating regular real-time current short pulse tests with data-driven Gaussian process regression algorithm, an efficient battery estimation has been successfully developed and validated for batteries with capacity ranging from 100% of the state of health (SOH) to below 50%, reaching an average accuracy as high as 95%. It remains challenging to effectively estimate the remaining capacity of the secondary lithium-ion batteries that have been widely adopted for consumer electronics, energy storage, and electric vehicles.
